Supervised Self Organizing Maps
The Problem
In a recent deterministic study carried out for a client it was concluded that the deterministic petrophysical summary simply was not credible. We concluded the evaluation was probably significantly underestimating the volume of sand in the formation system under scrutiny.
The solution
For that reason, it was decided to undertake an analysis based on the Machine Learning (ML) technique referred to as Self Organizing Map (SOM). The outcome is a completely revised understanding of the volume of sand in the reservoir. Moreover, it should significantly improve the information available from the wells 1 and well 2 for making a reservoir model for the prospect.
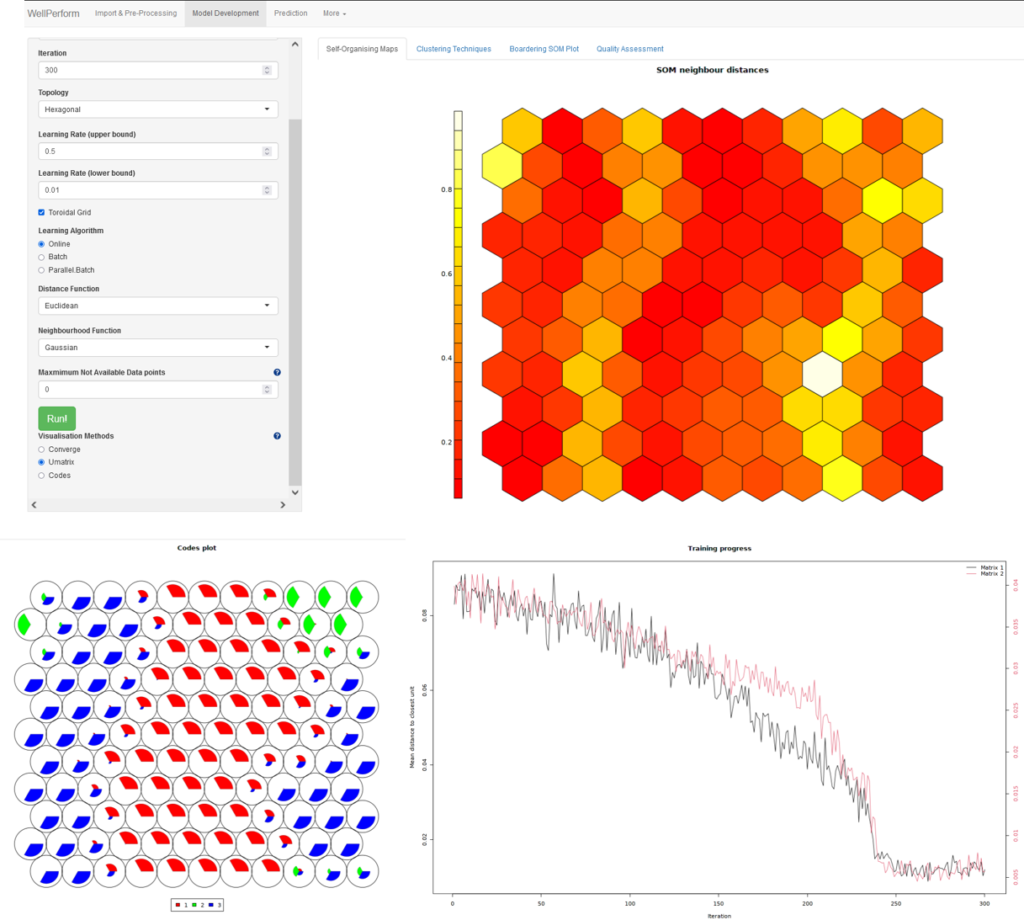
Scope of work.
- Facies Analysis from Core
- Improve the quantification of the amount of sand contained within the Sand 1 and Sand 2 intervals.
- Extract flow unit information using rock typing and extract beyond the cored intervals to both wells.
*For more information please check the original article here.
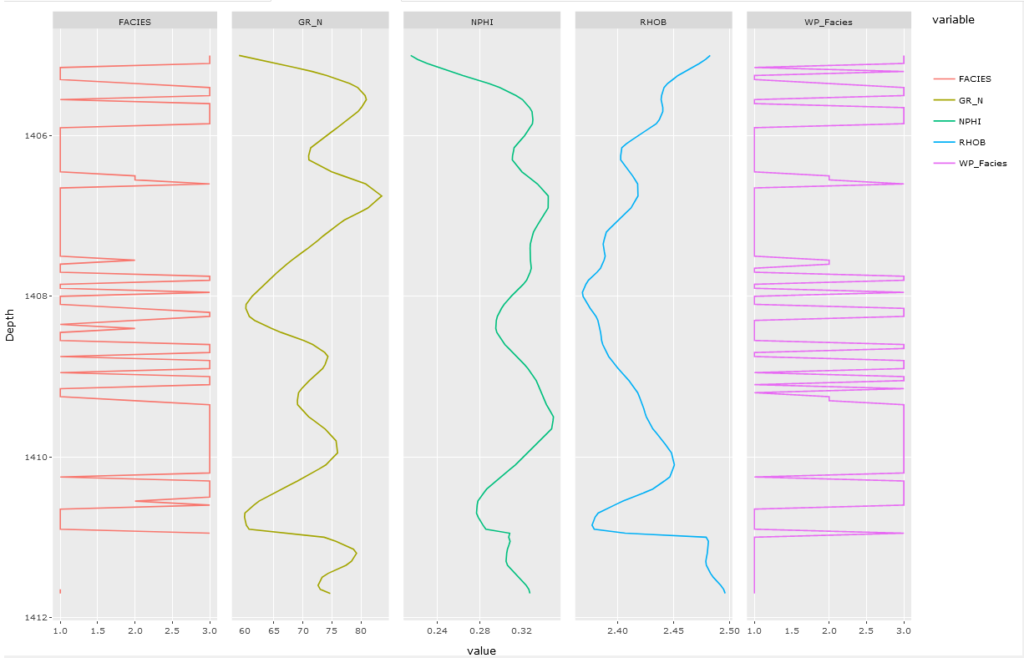
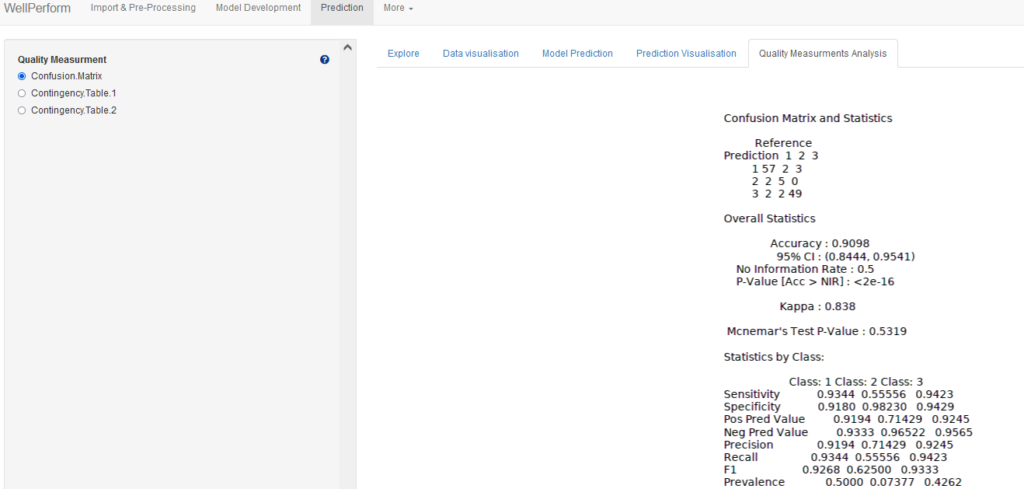